“ChatGPT can’t handle complex Jobs-to-be-Done Research”
We hear this all the time from professionals lamenting the arrival of a fierce competitor. I was right there with them a year ago. The competitor isn’t the AI itself, but the early adopters who are learning how to use it to their competitive advantage. I’ve been whacking away at this complexity since the end of 2022 and I’ve made progress. A lot of progress. There will be those who absolutely say it will never be as good as them, and I would suggest that their customers aren’t the ones I’m interested in (and they’re also wrong).
New business models will emerge that address more of the market. That is all 😉
My Experiment
Basically, AI solves for the average person, whether they be someone trying to conduct an interview or someone trying to respond to interview questions. People can only operate within their realm of experience and knowledge…so there are gaps. They can only communicate as well as they can communicate…so there are interpretation issues.
AI also solves for limited information access. We can put together the best screener and hire the best recruiting firm, but that is no guaranty that we can pull the perfect information we seek from the people we gain access to. For the qualitative part of Jobs-to-be-Done we're not looking for aha! insights, we’re looking for logical insights that help us to construct a model of the problem-space, including all of the possible ways that success could be measured.
I question any average person’s ability to do this perfectly. I question the superstars as well. After all, no one is perfect. Therefore, I’d like to share the process I’m currently using to develop the best possible models, as quickly and inexpensively as possible. Then we can prioritize them in the market to get the insights we seek.
The Job: Growing a Crop of Corn
Some of you may be familiar with Strategyn’s story around a farmer growing corn. As far as I know there is no case study associated with this. But, there are references to it in several PowerPoint decks that I’ve seen; one of which I found on the Internet over 10 years ago.
The deck has a job map for Farming Corn and states that there are 156 desired outcomes. Unfortunately, there were only 15 metrics shared in the deck, so we don’t have a lot to compare my experiment to.
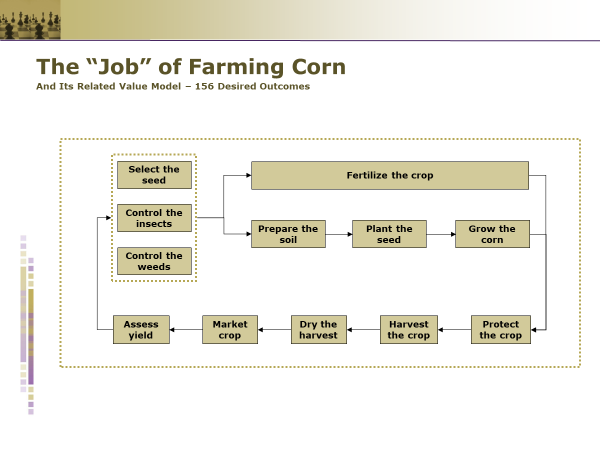
This was done a long time ago and the method has evolved. Job Maps are now linear and do not involve steps that are better suited as Related Jobs. Fertilize the crop is a potential example of this. Yea, this is kind of a weird job map.
Also, Strategyn migrated away from metrics that are better suited for consumption jobs in favor of Minimize the time it takes and Minimize the likelihood that/of. You’ll see those rules broken in the few example we have.
Note: All of the data I have used or generated in this experiment can be found in my Notion database. Direct link for paid subscribes at the end of this post 👇.
Here’s the Gumroad link (it’s still free, I just want your email) https://jtbd.one/growing-corn
I run through the experiment in more detail in the Notion database, but here’s the general idea:
Generate desired outcomes for the original job map to see how it compares (not much to compare it to for this one).
Generate 3 job maps with desired outcomes. I do this by using the same prompt and inputs several times on ChatGPT. I did this step-by-step, so as to optimize the available memory. The output is always slightly different (this is true with humans as well). The end user is a “farmer” and the job is “Growing a crop of corn”
I also generated two more map and outcome sets; one with ChatGPT and one with Claude.ai. I did this with a single prompt that generates the entire model in a single message. Admittedly, ChatGPT falls behind here a bit because of its memory/message length constraints. Claude is more powerful here, but I’m still evaluating the quality.
I then compare the maps side-by-side to spot the differences. Sometimes they are just wording, and other times there is a unique step added.
I create a strawman map (human powered) based on this comparison.
Experiment #1: I run my metrics prompt against the strawman to generate metrics for each step
Experiment #2: I synthesize the metrics across the different job map versions (across common steps) through ChatGPT to have it find overlaps and spot outliers. It then generates a new set derived from of the other sets it has produced.
Note: I decided not to do a human synthesis because the entire point here is to automate as much of this as possible.
I’ll let you all decide how well this did. Should we trust AI with Jobs-to-be-Done research? I’m pretty happy with the results.
As usual, I have developed a complete catalog which also includes
Situations (Complexity Factors)
Contexts
Use Cases
Related Jobs
Emotional Jobs
Social Jobs
Financial Metrics
And 150 Alternative metrics generated at the Job Level instead of the step level.
In my next experiment, I’ll start adding in my version of the metrics as I will demonstrate how powerful they are in getting to ultimate outcome being sought by end users. The topic? Optimizing Dairy Herd Productivity. That’s from a case study.
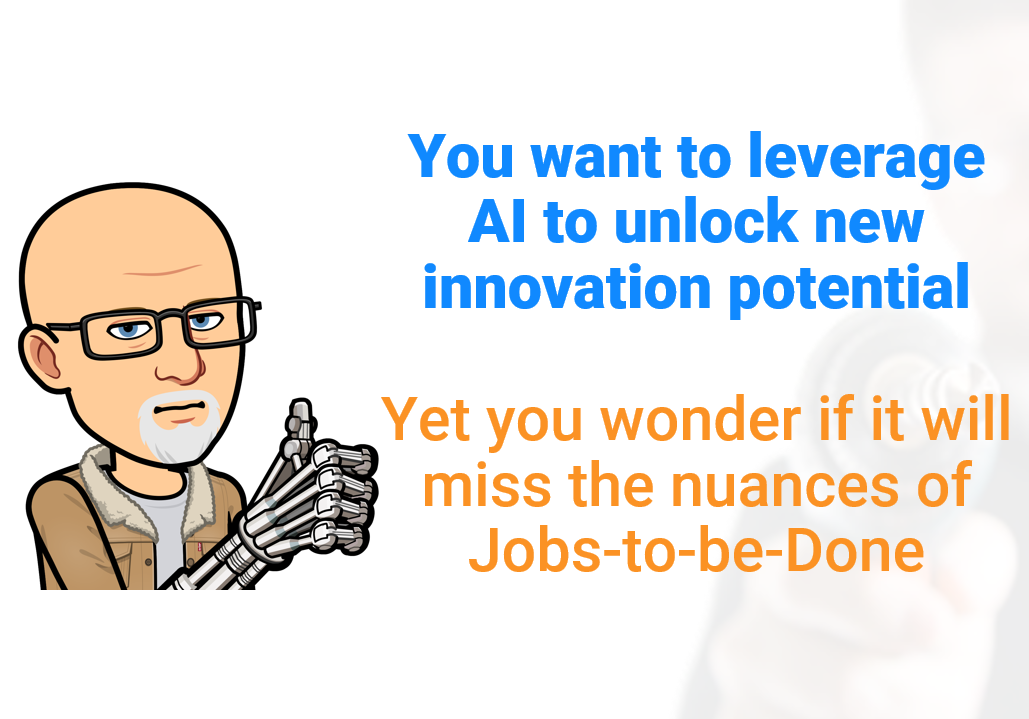
End the Analysis Paralysis
If you or your team is struggling with Jobs-to-be-Done, I’d like to help. Using my approach - with a little bit of human experience on top - I can help you to…
Quickly identify the right job to study
Quicky, accurately, and inexpensively generate a survey-worthy framework
Give you success metric formatting options that connect better with end users
If you need quantitative help, we can discuss that as well. It’s also faster, and way cheaper than the alternative options.
You can reach me at [email protected]